While deep learning (deep learning) educates machines to process data like the human brain, the so-called “black box” which hides the arbitrary predictions of algorithms artificial intelligence (AI) is increasingly worrying experts in this field.
The “black box” appears when the developers of these systems stop figuring out what’s going on in the alleys that the AI opens in its logical path, something hinders the control of one’s actions.
Many even fear that this lack of transparency could lead to irreversible consequencesespecially if this synthetic intelligence achieves skills for which it was not prepared or acquires total autonomy.
The alarm was raised weeks ago when a group of Google engineers were programming artificial intelligence software and discovered with surprise that, without warning, it had learned to connect a new language.
Google CEO, sundar pichaiindicated that this ability of AI programs to generate expertise or provide unexpected responses is what is known as “black box”.
Far from panicking, Pichai added, “Also, I don’t think we fully understand how the human mind works.” And he called specialists from different areas to discuss, in order to make the process less nebulous.
a path of no return
Some theorists understand that a tipping point has been reached where some types of AI have already surpassed others. the human mind. The problem is that the finite condition of man is incapable of embracing infinity: advanced AI.
An example of an “infinite” AI would be ChatGPT, which can write college-level essays and functional code. Plus, risk medical diagnoses, create text-based games, and explain scientific concepts in multiple levels of difficulty.
“Machine learning models are tested to determine if they work correctly and what is the degree of error they have. Since artificial intelligence systems are not infallible: the machine suggests a decision or a solution and the human being is the one who decides whether it is implemented”, he warns Marcella RiccilloPhD in computer science, expert in AI and robotics.
Unlike more traditional programming, which is based on implementing instructions to achieve a result, in AI development engineers work to build a system that mimics the “neural networks” of human intelligence.
Mathematical logic
In fact, deep learning algorithms are trained the same way a teacher explains a new concept to a child. Until he finally gets the idea.
Typically, examples of something you’re capable of recognizing are called, and before long your research proclivities will have built a “neural network” to classify things you’ve never experienced before.
“Some of the mathematical techniques of Machine Learning factor their results, such as decision trees. Neural networks, on the other hand, due to their enormous complexity, are not. In both cases we know what their structure is like, how they are internally and what the learning method is. Furthermore, in Neural Networks, the path to their conclusions is unknown and not even the results can be justified,” warns Riccillo.
As with human intelligence, there is little awareness of how a deep learning system reaches its conclusions. As Yoshua Bengio, a pioneer in this field, points out, “Once you have a sufficiently complicated machine, it becomes nearly impossible to explain what it does.”
The phenomenon of “black boxes” in AI is disturbing for the lack of understanding and control about how these systems acquire skills or provide answers in unexpected ways.
This situation raises ethical questions about the potential risks associated with the technology and its possible effects on a society defenseless against these cybernetic advances.
Pandora’s box of algorithms
The big challenge in this field is to develop techniques that justify the decision made by a machine learning algorithm without opening Pandora’s box.
But explaining AI decisions after they happen can have dangerous implications, says Cynthia Rudin, a computer science professor at Duke University.
“The neural networks, especially Deep Learning, used in ChatGPT are being questioned for not explaining the results. Several companies are trying to achieve this. But if an application with machine learning does not learn well, in each technique you can try to improve the model, even if it is not always possible. Whether or not they explain their findings,” says Riccillo.
Appealing to ChatGPT’s rote sincerity, this chronicler consulted him on the biases that the black box masks in generative AI.
“The black box is beyond human reach in AI systems that use complex algorithms, such as deep neural networks. Sometimes it can be difficult for people to understand how a specific decision was made, as there can be multiple layers of processing and calculation that are difficult to follow.”
These opaque models are catching on in some workplaces, and their spills are already leaving a trail of consequences. From approving a biopsy for a possible cancer, to issuing bail, bombing a military zone or approving a loan application.
Currently, approximately 581 models involved in medical decisions have received clearance from the Food and Drug Administration. Nearly 400 are intended to help radiologists detect medical imaging abnormalities, such as malignancies or signs of stroke.
Source: Clarin
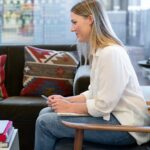
Linda Price is a tech expert at News Rebeat. With a deep understanding of the latest developments in the world of technology and a passion for innovation, Linda provides insightful and informative coverage of the cutting-edge advancements shaping our world.